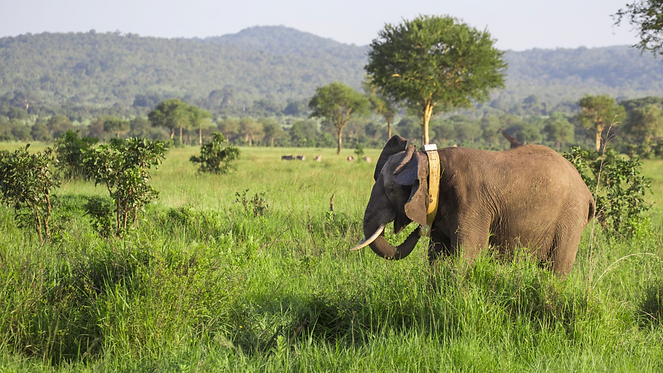
AMI - Automated Mammal Imagination
4.453 Creative Machine Learning
Professor Caitlin Mueller
With Joris Komen and Chili Cheng
The ability to understand and predict the complex interactions between wildlife species and their environments is crucial for effective conservation and wildlife management. This study aims to investigate these interactions by integrating topographic and land feature data with wildlife movement (GPS) data, and utilizing machine learning techniques to analyze patterns and predict how topographical or environmental changes may affect wildlife behavior. Our approach is multifaceted and attempts to find modal solutions for this complex problem. We utilize convolutional neural networks, Conditional Generative Adversarial Networks and Agent-Based Modeling, to find the embedded multidimensional relationships between 3D topographical information, land feature data and GPS tracking of animal movement.
Read our paper here.
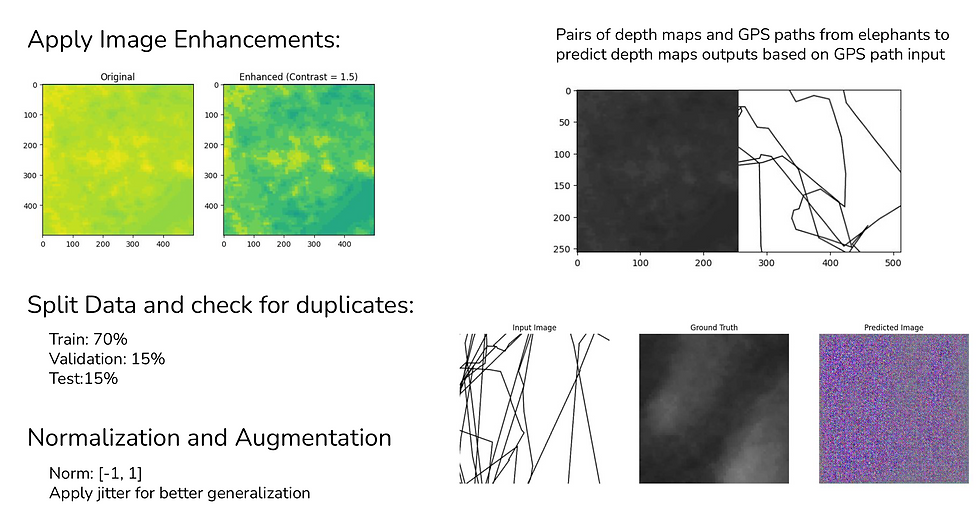
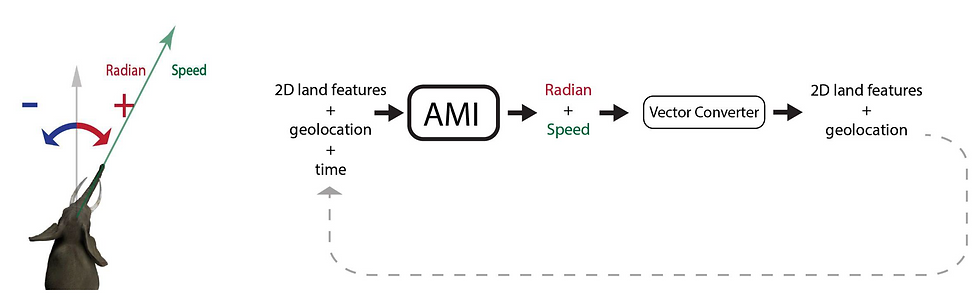
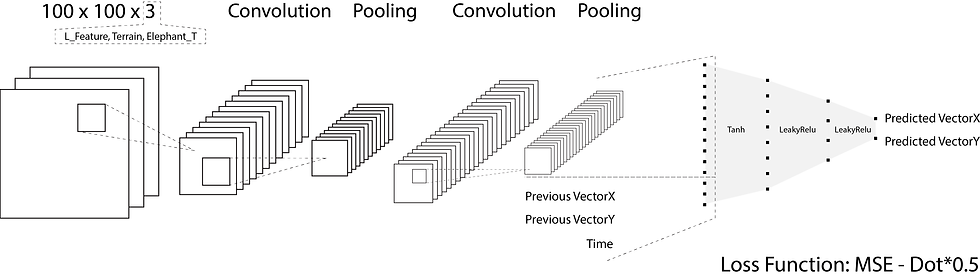